Use of artificial intelligence in CT image evaluation in stroke patients – current options
Authors:
Z. Trabalková 1,2; M. Števík 1; J. Sýkora 1,2; M. Vorčák 1; K. Zeleňák 1
Authors‘ workplace:
Rádiologická klinika JLF UK a UNM, Martin, SR
1; Rádiologická klinika LF UP a FN Olomouc, ČR
2
Published in:
Cesk Slov Neurol N 2024; 87(1): 32-40
Category:
Review Article
doi:
https://doi.org/10.48095/cccsnn202432
Overview
Artificial intelligence and its rapid development represent one of the most important technological advances of the current decade. It affects almost all aspects of life, including medicine. Artificial intelligence is widely applied in neuroradiology, particularly in stroke diagnosis. The primary purpose of its application in this area is to accelerate the interpretation process, increase diagnostic accuracy, and help to select the treatment strategy. Clinicians involved in the initial management of a stroke patient should be familiar with the technical principles and possible use of artificial intelligence in neuroimaging, and they should know the strengths and weaknesses of the technology. This article briefly presents methods of artificial intelligence used in visual data processing. The main goal of the publication is to present particular automated analyses used in the interpretation of diagnostic information taken from CT images. CT is the primary choice in stroke diagnostics for most medical departments. The presented analyses are a calculation of the ASPECT score and detection of a hyperdense artery sign from non-contrast CT scans, identification of large vessel occlusion and collateral score evaluation from CTA, and creation of perfusion maps from CT perfusion.
Keywords:
deep learning – machine learning – ischemic stroke – large vessel occlusion – artifi cial intelligence
Sources
1. Soun JE, Chow DS, Nagamine M et al. Artificial intelligence and acute stroke imaging. AJNR Am J Neuroradiol 2021; 42 (1): 2–11. doi: 10.3174/ajnr.A6883.
2. Wafa HA, Wolfe CDA, Emmett E et al. Burden of stroke in Europe: thirty-year projections of incidence, prevalence, deaths, and disability-adjusted life years. Stroke 2020; 51 (8): 2418–2427. doi: 10.1161/STROKEAHA.120.029606.
3. Chen W, Wu J, Wei R et al. Improving the diagnosis of acute ischemic stroke on non-contrast CT using deep learning: a multicenter study. Insights Imaging 2022; 13 (1): 184. doi: 10.1186/s13244-022-01331-3.
4. Kundeti SR, Vaidyanathan MK, Shivashankar B et al. Systematic review protocol to assess artificial intelligence diagnostic accuracy performance in detecting acute ischaemic stroke and large-vessel occlusions on CT and MR medical imaging. BMJ Open 2021; 11 (3): e043665. doi: 10.1136/bmjopen-2020-043665.
5. Shlobin NA, Baig AA, Waqas M et al. Artificial intelligence for large-vessel occlusion stroke: a systematic review. World Neurosurg 2022; 159: 207–220.e1. doi: 10.1016/j.wneu.2021.12.004.
6. Yahav-Dovrat A, Saban M, Merhav G et al. Evaluation of artificial intelligence-powered identification of large-vessel occlusions in a comprehensive stroke center. AJNR Am J Neuroradiol 2021; 42 (2): 247–254. doi: 10.3174/ajnr.A6923.
7. Lotan E. Emerging artificial intelligence imaging applications for stroke interventions. AJNR Am J Neuroradiol 2021; 42 (2): 255–256. doi: 10.3174/ajnr.A6902.
8. Mokli Y, Pfaff J, Dos Santos DP et al. Computer-aided imaging analysis in acute ischemic stroke – background and clinical applications. Neurol Res Pract 2019; 1: 23. doi: 10.1186/s42466-019-0028-y.
9. European Society of Radiology (ESR). What the radiologist should know about artificial intelligence – an ESR white paper. Insights Imaging 2019; 10 (1): 44. doi: 10.1186/s13244-019-0738-2.
10. Moawad AW, Fuentes DT, ElBanan MG et al. Artificial intelligence in diagnostic radiology: where do we stand, challenges, and opportunities. J Comput Assist Tomogr 2022; 46 (1): 78–90. doi: 10.1097/RCT.0000000000001247.
11. Kasalak Ö, Alnahwi H, Toxopeus R et al. Work overload and diagnostic errors in radiology. Eur J Radiol 2023; 167: 111032. doi: 10.1016/j.ejrad.2023.111032.
12. Muehlematter UJ, Bluethgen C, Vokinger KN. FDA-cleared artificial intelligence and machine learning--based medical devices and their 510 (k) predicate networks. Lancet Digit Health 2023; 5 (9): e618–e626. doi: 10.1016/S2589-7500 (23) 00126-7.
13. Olthof AW, van Ooijen PMA, Rezazade Mehrizi MH. Promises of artificial intelligence in neuroradiology: a systematic technographic review. Neuroradiology 2020; 62 (10): 1265–1278. doi: 10.1007/s00234-020-02424-w.
14. Lee EJ, Kim YH, Kim N et al. Deep into the brain: artificial intelligence in stroke imaging. J Stroke 2017; 19 (3): 277–285. doi: 10.5853/jos.2017.02054.
15. Chandrabhatla AS, Kuo EA, Sokolowski JD et al. Artificial intelligence and machine learning in the diagnosis and management of stroke: a narrative review of United States food and drug administration-approved technologies. J Clin Med 2023; 12 (11): 3755. doi: 10.3390/jcm12113755.
16. Monsour R, Dutta M, Mohamed AZ et al. Neuroimaging in the era of artificial intelligence: current applications. Fed Pract 2022; 39 (Suppl 1): S14–S20. doi: 10.12788/fp.0231.
17. Zaharchuk G, Gong E, Wintermark M et al. Deep learning in neuroradiology. AJNR Am J Neuroradiol 2018; 39 (10): 1776–1784. doi: 10.3174/ajnr.A5543.
18. Lee JG, Jun S, Cho YW et al. Deep learning in medical imaging: general overview. Korean J Radiol 2017; 18 (4): 570–584. doi: 10.3348/kjr.2017.18.4.570.
19. Chartrand G, Cheng PM, Vorontsov E et al. Deep learning: a primer for radiologists. Radiographics 2017; 37 (7): 2113–2131. doi: 10.1148/rg.2017170077.
20. Mazurowski MA, Buda M, Saha A et al. Deep learning in radiology: an overview of the concepts and a survey of the state of the art with focus on MRI. J Magn Reson Imaging 2019; 49 (4): 939–954. doi: 10.1002/jmri.26534.
21. Zeleňák K, Krajina A, Meyer L et al. How to improve the management of acute ischemic stroke by modern technologies, artificial intelligence, and new treatment methods. Life (Basel) 2021; 11 (6): 488. doi: 10.3390/life11060488.
22. Singh NM, Harrod JB, Subramanian S et al. How machine learning is powering neuroimaging to improve brain health. Neuroinformatics 2022; 20 (4): 943–964. doi: 10.1007/s12021-022-09572-9.
23. Vagal A, Saba L. Artificial intelligence in „code stroke“ – a paradigm shift: do radiologists need to change their practice? Radiol Artif Intell 2022; 4 (2): e210204. doi: 10.1148/ryai.210204.
24. Murray NM, Unberath M, Hager GD et al. Artificial intelligence to diagnose ischemic stroke and identify large vessel occlusions: a systematic review. J Neurointerv Surg 2020; 12 (2): 156–164. doi: 10.1136/neurintsurg-2019-015135.
25. Elijovich L, Dornbos Iii D, Nickele C et al. Automated emergent large vessel occlusion detection by artificial intelligence improves stroke workflow in a hub and spoke stroke system of care. J Neurointerv Surg 2022; 14 (7): 704–708. doi: 10.1136/neurintsurg-2021-017714.
26. Seker F, Pfaff J, Nagel S et al. CT reconstruction levels affect automated and reader-based ASPECTS ratings in acute ischemic stroke. J Neuroimaging 2019; 29 (1): 62–64. doi: 10.1111/jon.12562.
27. Sundaram VK, Goldstein J, Wheelwright D et al. Automated ASPECTS in acute ischemic stroke: a comparative analysis with CT perfusion. AJNR Am J Neuroradiol 2019; 40 (12): 2033–2038. doi: 10.3174/ajnr.A6303.
28. Maegerlein C, Fischer J, Mönch S et al. Automated calculation of the Alberta stroke program early CT score: feasibility and reliability. Radiology 2019; 291 (1): 141–148. doi: 10.1148/radiol.2019181228.
29. Bendszus M, FIehler J, Subtil F et al. Endovascular thrombectomy for acute ischaemic stroke with established large infarct: multicentre, open-label, randomised trial. Lancet 2023; 402 (10414): 1753–1763. doi: 10.1016/S0140-6736 (23) 02032-9.
30. Kuang H, Najm M, Chakraborty D et al. Automated ASPECTS on noncontrast CT scans in patients with acute ischemic stroke using machine learning. AJNR Am J Neuroradiol 2019; 40 (1): 33–38. doi: 10.3174/ajnr.A5889.
31. Nagel S, Sinha D, Day D et al. e-ASPECTS software is non-inferior to neuroradiologists in applying the ASPECT score to computed tomography scans of acute ischemic stroke patients. Int J Stroke 2017; 12 (6): 615–622. doi: 10.1177/1747493016681020.
32. Li X, Zhen Y, Liu H et al. Automated ASPECTS in acute ischemic stroke: comparison of the overall scores and Hounsfield unit values of two software packages and radiologists with different levels of experience. Acta Radiol 2023; 64 (1): 328–335. doi: 10.1177/02841851221075789.
33. Goebel J, Stenzel E, Guberina N et al. Automated ASPECT rating: comparison between the Frontier ASPECT Score software and the Brainomix software. Neuroradiology 2018; 60 (12): 1267–1272. doi: 10.1007/s00234-018-2098-x.
34. Hoelter P, Muehlen I, Goelitz P et al. Automated ASPECT scoring in acute ischemic stroke: comparison of three software tools. Neuroradiology 2020; 62 (10): 1231–1238. doi: 10.1007/s00234-020-02439-3.
35. Neuberger U, Nagel S, Pfaff J et al. Impact of slice thickness on clinical utility of automated Alberta Stroke Program Early Computed Tomography Scores. Eur Radiol 2020; 30 (6): 3137–3145. doi: 10.1007/s00330-019-066 16-8.
36. Amukotuwa SA, Straka M, Smith H et al. Automated detection of intracranial large vessel occlusions on computed tomography angiography: a single center experience. Stroke 2019; 50 (10): 2790–2798. doi: 10.1161/STROKEAHA.119.026259.
37. Barreira C, Bouslama M, Lim J et al. E-108 Aladin study: automated large artery occlusion detection in stroke imaging study – a multicenter analysis. J Neurointervent Surg 2018; 10 (Suppl 2): A101.2–A102. doi: 10.1136/neurintsurg-2018-SNIS.184.
38. Chung CY, Rodrigues GM, Haussen DC et al. Abstract WP76: automated detection of hyperdense MCA sign and automated notification of large vessel occlusion using artificial intelligence. Stroke 2019; 50 (Suppl 1): AWP76. doi: 10.1161/str.50.suppl_1.WP76.
39. Takahashi N, Lee Y, Tsai DY et al. An automated detection method for the MCA dot sign of acute stroke in unenhanced CT. Radiol Phys Technol 2014; 7 (1): 79–88. doi: 10.1007/s12194-013-0234-1.
40. Olive-Gadea M, Crespo C, Granes C et al. Deep learning based software to identify large vessel occlusion on noncontrast computed tomography. Stroke 2020; 51 (10): 3133–3137. doi: 10.1161/STROKEAHA.120.030326.
41. Rodrigues G, Barreira CM, Bouslama M et al. Automated large artery occlusion detection in stroke: a single-center validation study of an artificial intelligence algorithm. Cerebrovasc Dis 2022; 51 (2): 259–264. doi: 10.1159/000519125.
42. Seker F, Pfaff JAR, Mokli Y et al. Diagnostic accuracy of automated occlusion detection in CT angiography using e-CTA. Int J Stroke 2022; 17 (1): 77–82. doi: 10.1177/1747493021992592.
43. Wolff L, Su J, Van Loon D et al. Inter-rater reliability for assessing intracranial collaterals in patients with acute ischemic stroke: comparing 29 raters and an artificial intelligence-based software. Neuroradiology 2022; 64 (12): 2277–2284. doi: 10.1007/s00234-022-02984-z.
44. Tan IY, Demchuk AM, Hopyan J et al. CT angiography clot burden score and collateral score: correlation with clinical and radiologic outcomes in acute middle cerebral artery infarct. AJNR Am J Neuroradiol 2009; 30 (3): 525–531. doi: 10.3174/ajnr.A1408.
45. Rava RA, Seymour SE, Snyder KV et al. Automated collateral flow assessment in patients with acute ischemic stroke using computed tomography with artificial intelligence algorithms. World Neurosurg 2021; 155: e748–e760. doi: 10.1016/j.wneu.2021.08.136.
46. Grunwald IQ, Kulikovski J, Reith W et al. Collateral automation for triage in stroke: evaluating automated scoring of collaterals in acute stroke on computed tomography scans. Cerebrovasc Dis 2019; 47 (5–6): 217–222. doi: 10.1159/000500076.
47. Jabal MS, Kallmes DF, Harston G et al. Automated CT angiography collateral scoring in anterior large vessel occlusion stroke: a multireader study. Interv Neuro- radiol 2023; 15910199221150470. doi: 10.1177/159101 99221150470.
48. Wouters A, Robben D, Christensen S et al. Prediction of stroke infarct growth rates by baseline perfusion imaging. Stroke 2022; 53 (2): 569–577. doi: 10.1161/STROKEAHA.121.034444.
49. Copen WA, Yoo AJ, Rost NS et al. In patients with suspected acute stroke, CT perfusion-based cerebral blood flow maps cannot substitute for DWI in measuring the ischemic core. PLoS One 2017; 12 (11): e0188891. doi: 10.1371/journal.pone.0188891.
50. Muehlen I, Borutta M, Siedler G et al. Prognostic accuracy of CTP summary maps in patients with large vessel occlusive stroke and poor revascularization after mechanical thrombectomy-comparison of three automated perfusion software applications. Tomography 2022; 8 (3): 1350–1362. doi: 10.3390/tomography8030109.
51. Kasasbeh AS, Christensen S, Parsons MW et al. Artificial neural network computer tomography perfusion prediction of ischemic core. Stroke 2019; 50 (6): 1578–1581. doi: 10.1161/STROKEAHA.118.022649.
52. Bhurwani MMS, Boutelier T, Davis A et al. Identification of infarct core and ischemic penumbra using computed tomography perfusion and deep learning. J Med Imaging (Bellingham) 2023; 10 (1): 014001. doi: 10.1117/1.JMI.10.1.014001.
53. Gibson E, Georgescu B, Ceccaldi P et al. Artificial intelligence with statistical confidence scores for detection of acute or subacute hemorrhage on noncontrast CT head scans. Radiol Artif Intell 2022; 4 (3): e210115. doi: 10.1148/ryai.210115.
54. Schmitt N, Mokli Y, Weyland CS et al. Automated detection and segmentation of intracranial hemorrhage suspect hyperdensities in non-contrast-enhanced CT scans of acute stroke patients. Eur Radiol 2022; 32 (4): 2246–2254. doi: 10.1007/s00330-021-08352-4.
55. Heit JJ, Coelho H, Lima FO et al. Automated cerebral hemorrhage detection using RAPID. AJNR Am J Neuroradiol 2021; 42 (2): 273–278. doi: 10.3174/ajnr.A6926.
56. Seyam M, Weikert T, Sauter A et al. Utilization of artificial intelligence-based intracranial hemorrhage detection on emergent noncontrast CT images in clinical workflow. Radiol Artif Intell 2022; 4 (2): e210168. doi: 10.1148/ryai.210168.
57. O‘Neill TJ, Xi Y, Stehel E et al. Active reprioritization of the reading worklist using artificial intelligence has a beneficial effect on the turnaround time for interpretation of head CT with intracranial hemorrhage. Radiol Artif Intell 2020; 3 (2): e200024. doi: 10.1148/ryai.2020200024.
58. McLouth J, Elstrott S, Chaibi Y et al. Validation of a deep learning tool in the detection of intracranial hemorrhage and large vessel occlusion. Front Neurol 2021; 12: 656112. doi: 10.3389/fneur.2021.656112.
59. Voter AF, Meram E, Garrett JW et al. Diagnostic accuracy and failure mode analysis of a deep learning algorithm for the detection of intracranial hemorrhage. J Am Coll Radiol 2021; 18 (8): 1143–1152. doi: 10.1016/ j.jacr.2021.03.005.
60. Mair G, White P, Bath PM et al. External validation of e-ASPECTS software for interpreting brain CT in stroke. Ann Neurol 2022; 92 (6): 943–957. doi: 10.1002/ana.26495.
Labels
Paediatric neurology Neurosurgery NeurologyArticle was published in
Czech and Slovak Neurology and Neurosurgery
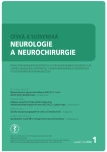
2024 Issue 1
Most read in this issue
- Diffuse glioma overview based on the 2021 WHO classifi cation part 2 – pediatric type
- Tonsilla cerebelli – anatomy, function and its significance for neurosurgery
- Standardization of MRI in Multiple Sclerosis Management Consensus by the Czech Expert Radiology-Neurology Panel
- Adaptation and validation of the Czech version of the Addenbrooke‘s Cognitive Examination (ACE-III-CZ) – pilot study